Trending Research Topics in Data Science
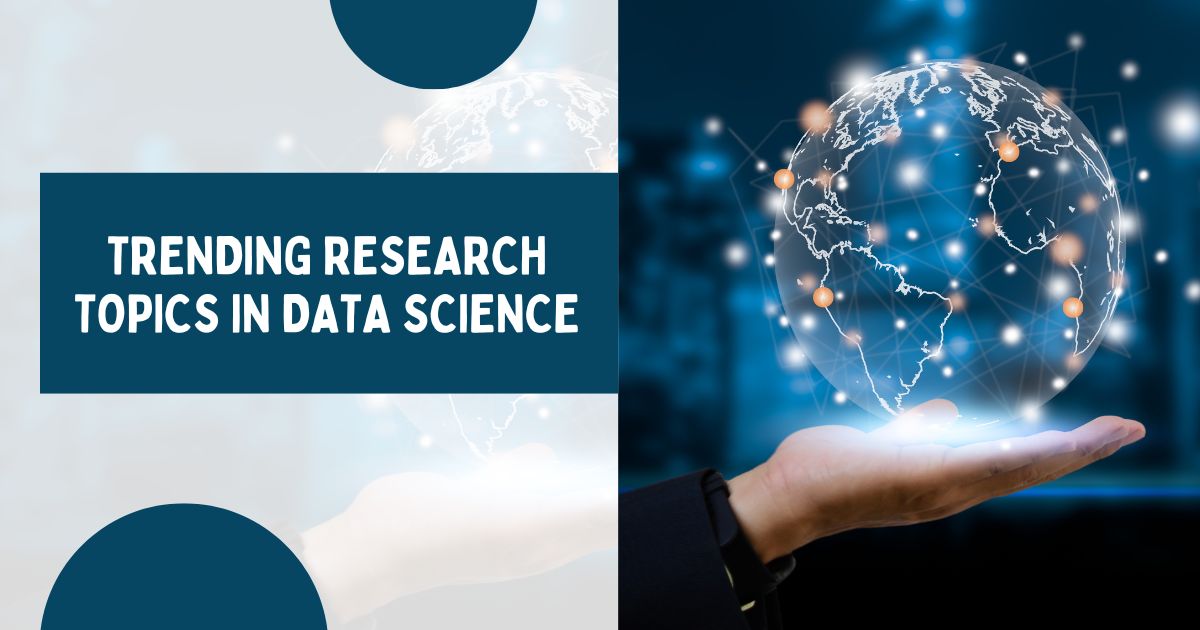
Data science has evolved rapidly over the past decade, transforming industries from healthcare to finance, and even entertainment. As technology progresses, new challenges and opportunities continually arise in the field. Some of the most exciting and trending research topics in data science today are at the intersection of machine learning, artificial intelligence, big data, and computational methods. Here are some of the key areas of active research in data science.
1. Artificial Intelligence and Machine Learning Advancements
Artificial Intelligence (AI) and Machine Learning (ML) are core components of modern data science. As algorithms become more sophisticated, there is an increasing focus on advancing these techniques to make them more interpretable, efficient, and applicable to a wider range of problems. In particular, deep learning continues to be an area of significant interest, especially in the context of unsupervised learning and reinforcement learning. Researchers are exploring ways to reduce the need for large labeled datasets, improve generalization in models, and optimize neural networks to make them more resource-efficient.
Explainable AI (XAI) is another prominent area. As AI systems become more embedded in critical applications, such as healthcare or finance, it is essential to ensure that decisions made by these models are interpretable and understandable by humans. Research in XAI focuses on creating algorithms that not only make accurate predictions but also provide insights into how those predictions were made, which is crucial for transparency, trust, and ethical concerns.
2. Automated Machine Learning (AutoML)
Automated Machine Learning (AutoML) aims to make machine learning more accessible to non-experts while improving the productivity of data scientists. The research in AutoML focuses on developing systems that can automatically choose, train, and fine-tune machine learning models. Key topics include hyperparameter optimization, neural architecture search, and automated feature engineering. This research is designed to make data science more efficient and help organizations deploy machine learning models without requiring extensive expertise in the field.
3. Data Privacy and Security
As data collection becomes more pervasive, ensuring the privacy and security of sensitive information is a critical concern. Research in this area revolves around differential privacy, federated learning, and cryptographic techniques for data protection. Differential privacy, for instance, involves techniques that allow data to be analyzed while ensuring that the privacy of individuals is not compromised. Federated learning enables machine learning models to be trained across decentralized devices or servers without raw data leaving the devices, which helps in reducing the risk of data breaches. This area is particularly important for industries like healthcare, finance, and social media, where user privacy is a major concern.
4. Natural Language Processing (NLP)
Natural Language Processing continues to be a thriving field in data science. With the rise of large language models like GPT and BERT, research is focused on improving the efficiency and capabilities of NLP systems. A significant area of study is multi-modal learning, where models integrate text, images, and other forms of data to improve understanding. Another area of focus is zero-shot learning—the ability of models to perform tasks they have never been explicitly trained on. Additionally, researchers are working on developing more sophisticated sentiment analysis, text summarization, and translation techniques, making NLP even more powerful and adaptable.
5. Ethics and Bias in Data Science
As machine learning models are increasingly deployed in real-world scenarios, concerns over algorithmic bias and ethical implications of automated decision-making have risen. Research is actively addressing issues such as biased datasets, discrimination in AI systems, and fairness in machine learning algorithms. Various frameworks and methods are being proposed to make AI models fairer and more equitable, such as adversarial debiasing, fairness-aware learning, and using counterfactual analysis to evaluate model decisions.
6. Quantum Computing and Data Science
Quantum computing is another emerging area of interest for data scientists. While still in its early stages, quantum computing has the potential to revolutionize data processing by enabling faster computations for certain types of problems, such as optimization and complex simulations. Research in this area is focused on developing quantum algorithms for machine learning, data analysis, and encryption, and understanding how quantum systems can be integrated with classical data science tools.
7. Edge Computing and Real-Time Analytics
As the Internet of Things (IoT) and connected devices continue to grow, there is an increasing demand for edge computing, where data is processed closer to its source, reducing latency and bandwidth usage. Research in edge computing is focused on real-time data analytics and the development of lightweight machine learning models that can run on devices with limited computational resources. This is particularly useful in applications like autonomous vehicles, smart cities, and industrial automation.
Conclusion
Data science continues to be one of the most dynamic and fast-evolving fields in technology. As we move forward, key research topics in data science such as AI and ML advancements, data privacy, ethics, and real-time analytics will shape the future of data science. The intersection of these areas promises to drive innovation across industries and tackle some of the most pressing challenges of the modern world. For those interested in contributing to this ever-growing field, staying informed about these research trends is essential.
Top Research Topics
https://topresearchtopics.com/
At Top Research Topics, we began with a simple idea: to make knowledge more accessible and relevant in a rapidly changing world. Founded by a team of passionate researchers and industry experts, we saw the need for a platform that could bridge the gap between academic research and real-world application.
Most Popular
- Methylcobalamin B12 Injections: Boosting Energy and Health
- Born X Raised: Redefining Streetwear with Authentic Style
- Revolutionizing Indian Education: A Closer Look at Modern Universities and Their Role in Shaping Future Leaders
- The Impact Of Social Media Marketing For E-Commerce Growth
- Why Businesses Consider SMD LED Screen Display for Advertising?
- Securing Aquarium During Home Shifting with top Packers and Movers in Vadodara
0 Comments
No Comments Found
LEAVE A REPLY
Your email address will not be published